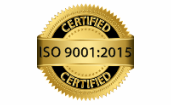
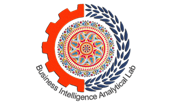
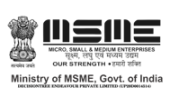
Quantitative tools such as modelling in the Financial Industry (Methods & Model) have been widely adopted in order to extract the massive information from a variety of financial data. Mathematics, statistics and computers algorithms are used for
Investment banks build up symmetry or equilibrium models to appraise financial instruments; mutual funds applied to time series to identify the risks in their portfolio; and hedge funds hope to extract market signals and statistical arbitrage from noisy market data. The rise of quantitative finance in the last decade relies on the development of computer programming oriented techniques that makes processing large datasets possible. As more data is available at a higher frequency, more researches in quantitative finance have switched to the microstructures of the financial market. High-frequency data is a typical example of big data that is characterized by the 3V’s: velocity, variety and volume.
Moreover, the indication or sign to noise ratio in monetary financial time series is usually exceptionally small. High-frequency datasets are more likely to be exposed to extreme values, missing value, jumps and errors than the low-frequency ones. Specific precise data processing techniques and quantitative models are elaborately designed to extract meaningful insight or information from financial data efficiently.